Track 2: Action Recognition from Dark Videos
Register for this track
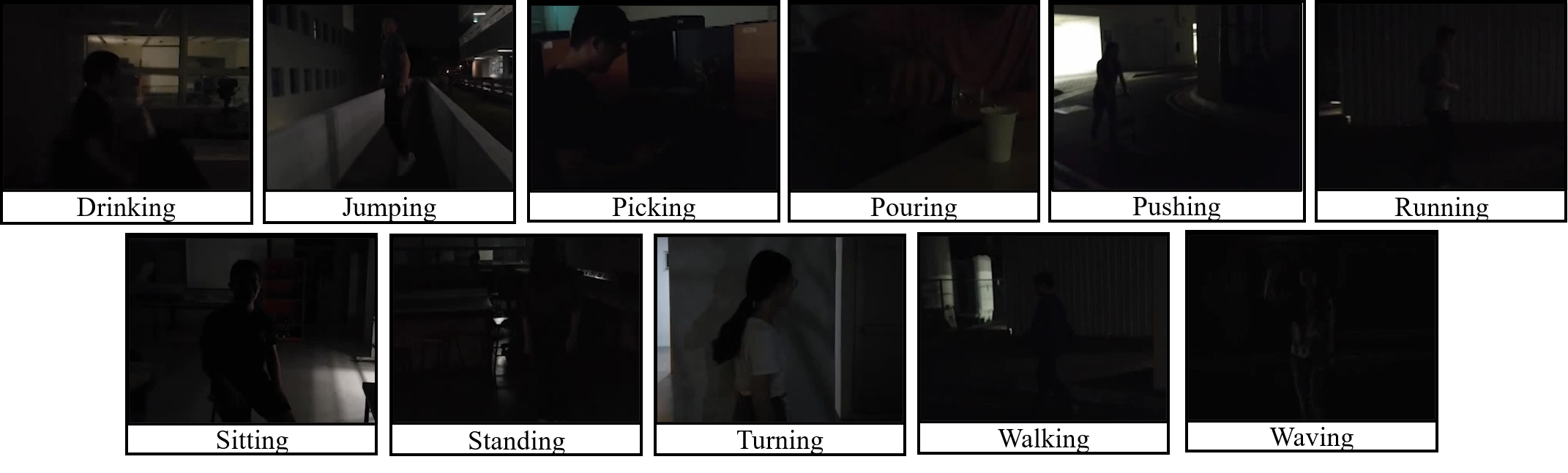
With the advances in computer vision technologies, especially video-based technologies, various automatic video-based tasks have received considerable attention. There have been increasing applications of these technologies in various fields, which include surveillance and smart homes. The advances can be credited in part to a rising number of video-based datasets, designed for a range of video-based tasks such as action recognition, localization and segmentation. Although much progress has been made, the progress is mostly limited to videos shot under “clear” environments, with normal illumination and contrast. Such limitation is partly due to the fact that current benchmark video-based datasets are collected from either crowdsourcing platforms or from public web video platforms directly. Either source contains mostly “clear” videos, where the actions are identifiable. However, there has been an increasing number of scenarios where videos shot under normal illumination may not be available. One notable scenario is night security surveillance, where security cameras are usually placed at alleys or fields with little lighting. The actions captured are hard to identify even by the naked eye. Although additional sensors, such as infrared imaging sensors, could be utilized for recognizing action in these environments, their cost prohibits the large scale deployment of these sensors. It is therefore greatly beneficial to explore possible video-based technologies that are robust to darkness, extracting effective action features from dark videos, which would benefit in various downstream applications.
Over the past few years, there has been a remarkable rise of research interest with regards to computer vision tasks in dark environments, which include dark face recognition and dark image enhancement. More recently, such research interests have been expanded to the video domain, especially towards darkness enhancement. However, it is noted that under image domain, visually enhanced dark images may not result in better classification accuracies when the same techniques are applied. Therefore it seems uncertain if the visually enhanced videos could necessarily result in better action recognition accuracies. Furthermore, with massive publicly available clear videos, it also seems promising to utilize them in some way, with possible pre-processing or post-processing steps, to capture actions with high robustness towards darkness.
UG2+ Challenge 2 aims to promote video-based action recognition algorithms’ robustness with special focus on dark videos via two different approaches represented by the following two sub-challenges:
- Fully Supervised Action Recognition in the Dark
- Semi-supervised Action Recognition in the Dark
In all two sub-challenges, the participant teams are allowed to use external training data that are not mentioned above, including self-synthesized or self-collected data; but they must state so in their submissions ("Method description" section in Codalab). The ranking criteria will be the Top-1 accuracy on each testing set.
References:
[1] Xu, Y., Yang, J., Cao, H., Mao, K., Yin, J. and See, S., 2020. ARID: A New Dataset for Recognizing Action in the Dark. arXiv preprint arXiv:2006.03876.
[2] Jhuang, H., Garrote, H., Poggio, E., Serre, T. and Hmdb, T., 2011, November. A large video database for human motion recognition. In Proc. of IEEE International Conference on Computer Vision (Vol. 4, No. 5, p. 6).
Footer